The Toronto Blue Jays, Tipping Pitches, and Artificial Intelligence
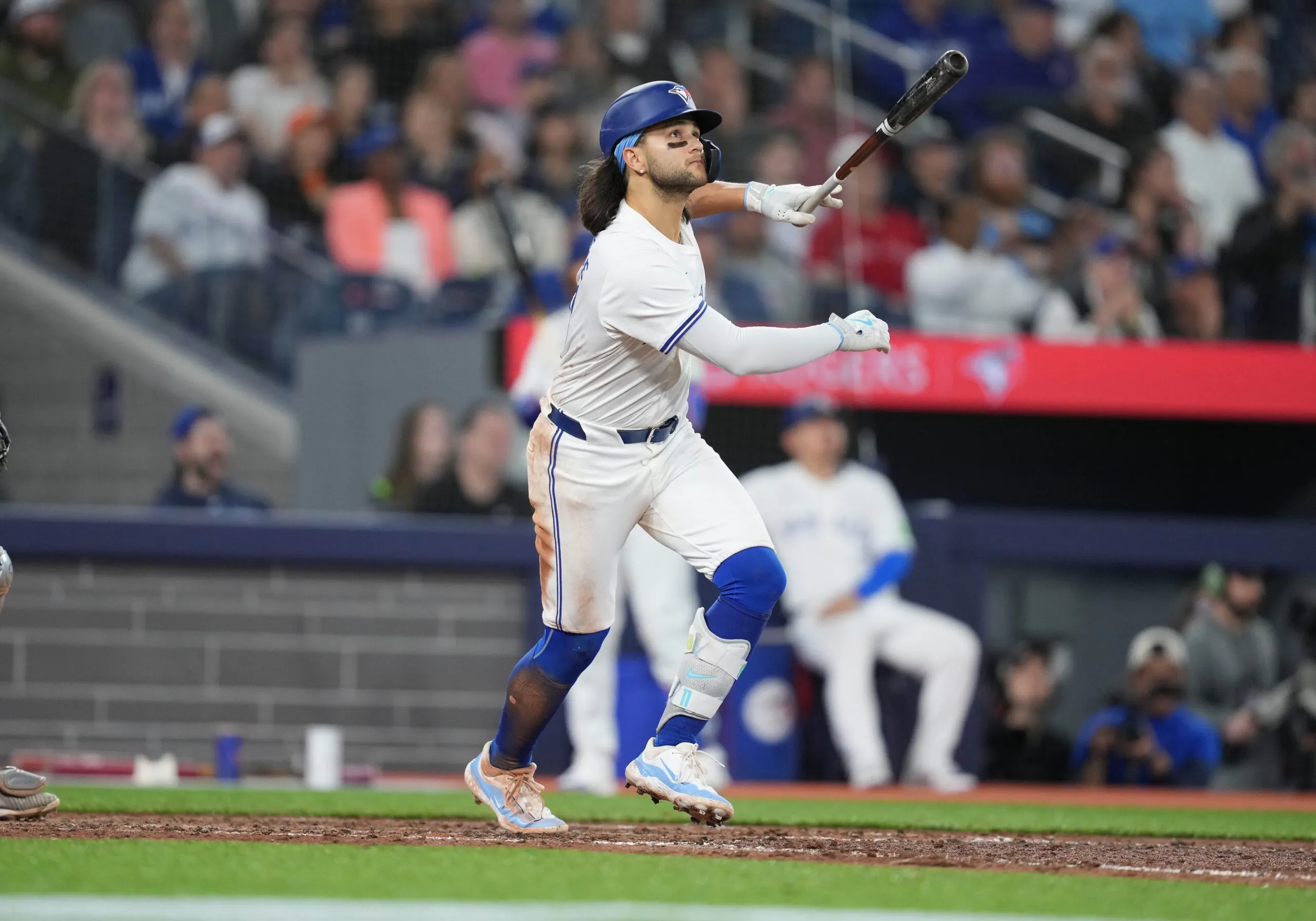
By Jim Scott
8 days agoIn a recent broadcast, Michael Kay, a New York Yankee announcer, wondered aloud how the Jays managed to improve so much from their first series in The Bronx a couple of weeks ago to the series in Toronto that just finished.
“You know what’s curious to me, though? We just saw the Blue Jays in The Bronx, and what we watched was a completely different team than the one we’ve seen here in Toronto. The one in New York did not foul off the amount of pitches this team does — it’s like it’s a different team in this ballpark. Now, home field advantage, there is something to that, but why would it manifest itself in the ability to foul off tough pitches? … You can understand it if the next time you see them, after you saw them in The Bronx, is a month and a half later, but this is a week later, and it’s a completely different approach and a completely different team.”
The implication was that the Jays were somehow anticipating the Yankee pitches better. The usual suspicion would be pitch tipping — but in this series, the Jays were hitting multiple New York pitchers better. Toronto could not have identified “tells” in multiple Yankee pitchers in such a short time.
Or could they?
An ex-MLB pitcher recently posted a video suggesting that artificial intelligence, compared with modern high-definition video feeds, could allow teams to perform much faster—and much more comprehensive and efficient — analyses of pitchers’ motions and habits. Teams are using technology more than ever, looking for an “edge.” Might Toronto — or some other teams — have computerized the search for pitch tells?
Tipping pitches
The idea of pitchers tipping pitches — and of opposing teams trying to identify those “tells” — is nothing new. “The Baseball Codes” identifies several examples:
When Babe Ruth first came to the American League as a pitcher with the Red Sox, he curled his tongue in the corner of his mouth whenever he threw a curveball—a habit he was forced to break once enough hitters became aware of it. Kansas City’s Mark Gubicza was cured of his tendency to stick out his tongue when throwing a breaking ball under similar circumstances. Ty Cobb regularly stole bases against Cy Young, abetted, said the outfielder, by the fact that Young’s arms drifted away from his body when he came set before throwing to first; when he was preparing to pitch, he pulled his arms in.
Tips could take many forms. A pitcher might grind his teeth for one pitch, grip the ball differently, or hold their head at a different angle. They might stand in a slightly different place on the rubber, dig the ball harder into their glove, or even do something as subtle as the position of one finger outside the glove. And tipping is not just ancient history. As author Jason Turbow notes:
Houston has recent history with this sort of strategy, winning the 2017 World Series after Carlos Beltran noticed that when Dodgers starter Yu Darvish re-gripped the ball while bringing it to his glove, he gave away whether he was about to throw a fastball or a breaking pitch. Darvish faced the Astros twice in the Series, throwing a total of 48 sliders and cutters, against which Houston batted .556. He didn’t make it out of the second inning either time, giving up five runs over 1.2 innings in the deciding Game 7
So, where does AI come in?
But up to now, identifying pitcher tells has been a manual exercise. Batters and coaches would observe pitchers, both during the game and in post-game videos, and look for these giveaways. This is similar to how teams worked with their hitters in the past, relying primarily on human review. But this approach has evolved, placing greater emphasis on technology like Rapsodo, HitTrax, and Trackman. These tools can process data at a much more detailed level and with greater accuracy than any human eye.
Might the same approach work for pitch tipping?
Imagine a heuristic AI “TipBot” algorithm that examines video of pitchers, looking for correlations. Such a program would have many advantages. It would be able to look at an extreme level of detail. Does the pitcher flare his nostrils before throwing a slider? Does he bend one knee slightly more for a curve? Is his release time one second faster for a four-seamer? This bot could also monitor literally thousands of things for each pitch – far more than a human could. And a bot could be completely objective, with none of the biases (confirmation bias? Halo effect? Availability Heuristic?) that a human observer might have.
Is it legal? Is it moral?
Under MLB rules, teams are not allowed to use video during an at-bat. But players can view an at-bat on their iPads once they are complete (the videos are edited to eliminate catcher signals, but that is less critical with PitchCom). In theory, teams could review similar videos of pitchers after each at-bat. And at AI processing speeds, anomalies could be identified almost instantly. So if it were a very hot day, and the pitcher (who normally has no tells at all) is wiping the perspiration differently for a breaking ball, the AI bot could identify and communicate it quickly enough for the team to take immediate advantage.
Of course, even if a team did not use the AI TipBot during the game, it could still be used after the game (or in advance of a particular series once a team knows which starters they would be facing). Teams are allowed to perform such evaluations under MLB rules, and there is no general prohibition against using technology.
The question of whether it is “fair” is more complex. The use of these bots by a team to evaluate their own pitchers, to ensure that other teams are not taking advantage. might generally be accepted. But is it fair to use technology to identify weaknesses that might not be readily visible to the human eye? Or trends that are not absolute (“if Pitcher X glances at third base, there is a 73% chance he will be throwing a slider”)? Or tells based on multiple factors (“if Pitcher X takes a deep breath and shakes his pitching hand, he will never throw to first base so it is safer to steal”) that a human might not put together?
The problem with fairness is consistency. It would be very difficult for MLB to effectively ban AI TipBots. The work could so easily be done remotely, and untraceably. And if one team is doing it to their advantage, but other teams are not, it would create an unfair imbalance. So would it be preferable for MLB to impose limits (like they did with Stickum) to ensure a level cyber playing field?
The bottom line
Teams are constantly looking for an advantage. Having a better understanding of the opposing pitchers could be a major advantage. So the question is not “Would teams use AI?” or even “Should teams use AI?” The more realistic question is likely “Are teams using AI TipBots right now?” and “What should our team be doing to stay ahead of that curve?”
Recent articles from Jim Scott